Behavioral segmentation of customers using math AI models
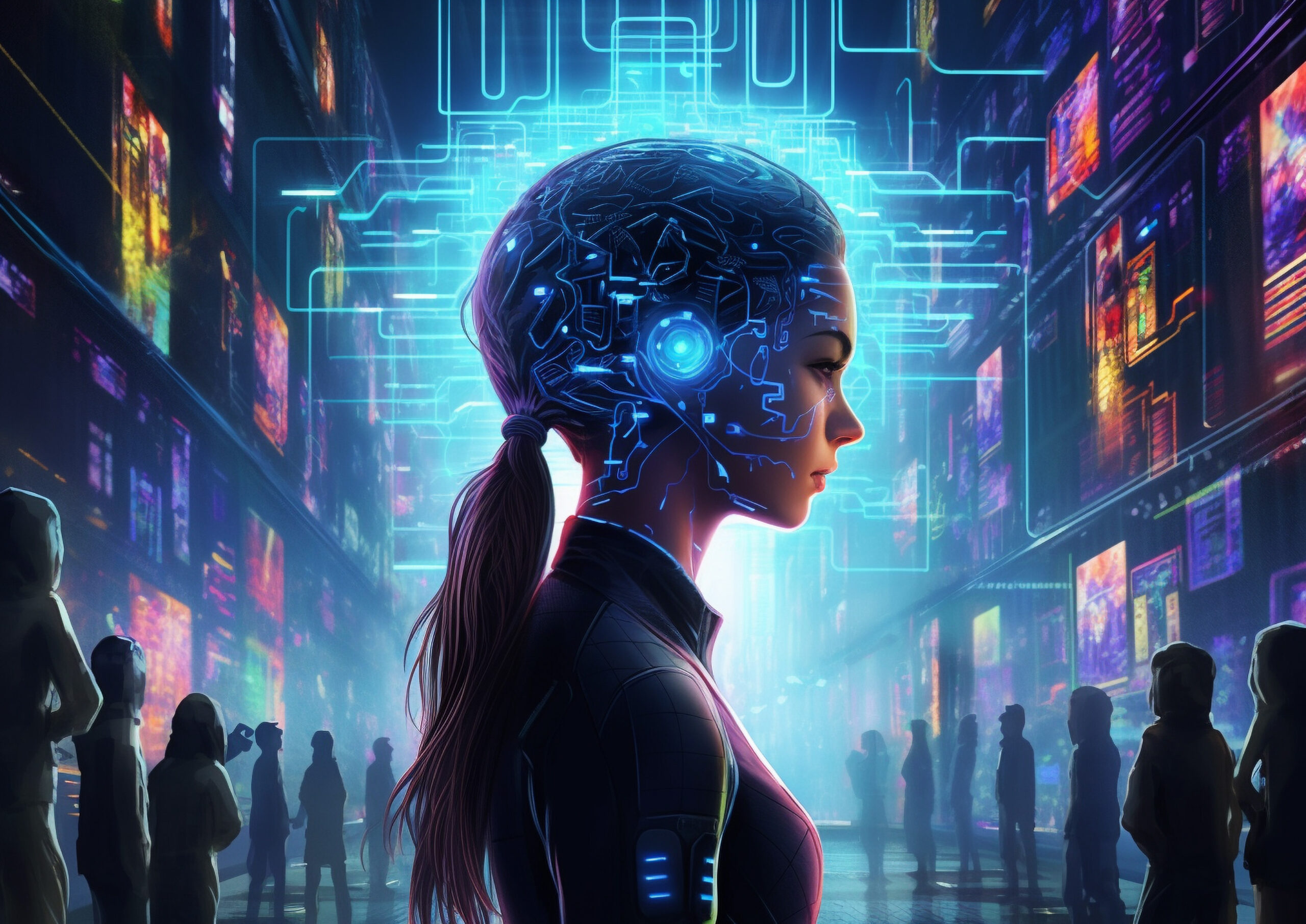
Understanding customers is a cornerstone of business success. Companies invest countless resources to decode consumer behavior, preferences, and tendencies. Behavioral segmentation, a strategy that groups customers based on their actions rather than demographics, is a powerful tool for achieving this. The advent of mathematical AI models has revolutionized this process, transforming it from a labor-intensive exercise into a streamlined, data-driven strategy. Let’s dive into how these innovations are reshaping the segmentation of customers.
The Basics of Behavioral Segmentation
Behavioral segmentation involves dividing a customer base into distinct groups based on their actions, such as purchasing habits, website interactions, or brand loyalty. Instead of asking, “Who is our customer?” the focus shifts to, “What does our customer do?”
For example, one group might consist of frequent buyers who shop during sales, while another could include occasional high-value spenders. Identifying these patterns allows businesses to tailor their marketing efforts. Research shows that companies using segmentation techniques experience a 10-15% increase in sales compared to those that don’t.
Traditional methods relied heavily on manual analysis and surveys. However, these techniques often fail to capture the nuance of real-time customer actions. Enter math AI models.
The Role of Math AI Models in Customer Segmentation
Mathematical AI models—ranging from neural networks to clustering algorithms—excel at analyzing complex data sets. These models process millions of data points to uncover patterns that humans might overlook.
Take k-means clustering, a popular method in behavioral segmentation. This algorithm groups customers into clusters based on shared traits. For instance, customers who frequently visit a website but rarely make purchases might form one cluster, while those who make regular, small transactions might belong to another. By mapping such behaviors, companies can refine their approaches to engagement.
Predictive analytics is another game-changer. Using historical data, AI models forecast future behaviors with remarkable accuracy. You can even identify certain patterns yourself if you are ready to work with a large amount of data. With the advent of AI solver, this will not be a problem. It will help you master algebra and calculus at a professional level. Most importantly, the AI helper app makes calculations fast, accurate and step-by-step. If something lies beyond the basic AI algorithm, now you can do it. A report by Statista estimates that by 2025, predictive analytics in customer segmentation will save businesses up to 30% on marketing costs.
The Math Behind AI-Driven Behavioral Segmentation
How do these models actually work? At the core of most segmentation strategies are mathematical principles like probability, statistics, and calculus. AI models leverage these foundations to make sense of data.
- Data Collection: The process begins with gathering customer data—purchase history, online behavior, email interactions, etc. This data serves as the raw material for segmentation.
- Feature Engineering: Once data is collected, AI models identify relevant features. For example, the frequency of purchases, average transaction value, and time spent on a website could all be critical variables.
- Segmentation Algorithms: Algorithms like Gaussian Mixture Models (GMM) or hierarchical clustering take over. GMM assumes data follows a probabilistic distribution and assigns customers to segments based on probabilities. Hierarchical clustering, on the other hand, builds a tree of clusters, making it easier to visualize relationships between groups.
- Validation and Iteration: The final step involves validating the model’s output. Businesses might test a segment-specific campaign to evaluate its effectiveness, iterating on the model to refine accuracy.
Real-World Applications
AI-driven behavioral segmentation isn’t just theoretical—it’s actively shaping industries.
- E-Commerce: Online retailers use AI models to identify and target high-value customers. For instance, a customer segment might include those who browse luxury items but abandon their carts. Personalized promotions can then nudge these customers toward completing their purchases.
- Streaming Services: Platforms like Netflix rely on behavioral segmentation to recommend content. By analyzing viewing history, AI models predict what users are likely to enjoy, increasing retention rates.
- Banking and Finance: Banks segment customers based on spending behaviors, identifying those likely to need loans or premium services. This approach has contributed to a 20% increase in cross-sell rates, according to a recent study by McKinsey.
Challenges and Ethical Considerations
Despite its benefits, behavioral segmentation isn’t without challenges.
- Data Quality: Poor data leads to poor results. Businesses must ensure their data is accurate, complete, and free from bias.
- Privacy Concerns: The rise of AI-driven segmentation has heightened data privacy concerns. Customers are increasingly wary of how their data is used. Ensuring compliance with regulations like GDPR and CCPA is non-negotiable.
- Over-Segmentation: Segmenting too narrowly can dilute marketing efforts, leading to inefficiencies. Striking the right balance is key.
The Future of Behavioral Segmentation
The integration of advanced math AI models into behavioral segmentation is still in its early stages, but the potential is staggering. As technology evolves, so will its applications.
- Real-Time Personalization: AI models are expected to deliver even more dynamic insights, enabling real-time adjustments to marketing strategies. Imagine walking into a store and receiving personalized offers on your phone based on your browsing history—this is no longer science fiction.
- Cross-Channel Integration: Future segmentation strategies will seamlessly merge online and offline behaviors. A customer’s in-store purchase could instantly update their online profile, creating a unified view of their journey.
- Enhanced Predictive Power: As AI continues to learn, its predictive capabilities will improve. Businesses will not only understand what customers want but also anticipate their needs before they arise.
Conclusion
Behavioral segmentation, powered by math AI models, represents a paradigm shift in how businesses engage with their customers. By leveraging advanced algorithms, companies can uncover actionable insights that were previously buried in mountains of data.
The key lies in embracing these technologies responsibly. With the right balance of innovation, ethical considerations, and strategic implementation, businesses can not only enhance their bottom line but also build lasting, meaningful relationships with their customers.
It’s not just about understanding customers anymore; it’s about predicting them—and math AI models are making that possible.
Miss Clipping Out Stories to Save for Later?
Click the Purchase Story button below to order a print of this story. We will print it for you on matte photo paper to keep forever.